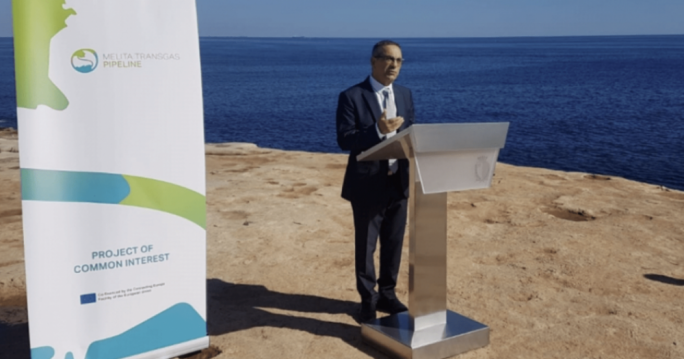
The end of data science as we know it?
Thus, Data Scientists should adopt adaptive governance, a flexible, agile decision-making process that allows fast responses while reducing risks
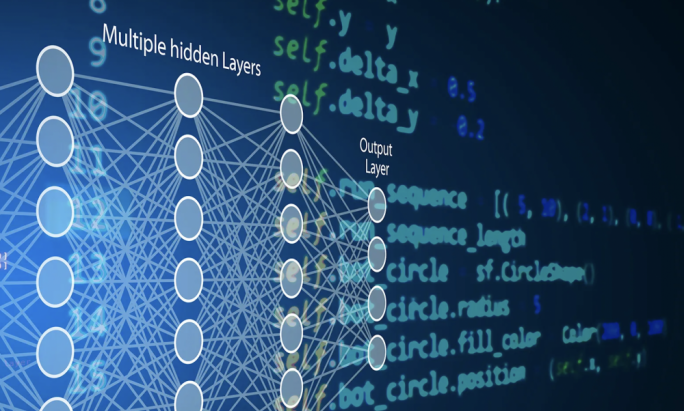
Data science (DS) is a field of study which uses advanced analytical techniques to extract valuable information from data. To do so, it utilizes Statistics, Artificial Intelligence (AI), Mathematics, and a bag load of other techniques. These insights are then used by businesses for decision-making and strategic planning.
Its roots can be traced back to the 80s, but DS gained massive popularity among many professionals in the last decade. With the proliferation of digital transformation amongst different organizations, the availability of cheap processing power, and the development of powerful algorithms, DS became accessible to almost anyone who needs it. However, in the past few years, it has become evident that Data Science is going through a transformation process.
First of all, people are drowning in data but thirsting for information. With the advancement of digitization and the proliferation of Internet-of-Things (IoT) devices, this is becoming increasingly true. Unfortunately, the tsunami of data has led Data Scientists to become data plumbers gathering all the data they can and sticking it into the system. But such an approach is not feasible and will become unmanageable in the long run because it will impact the system’s scalability. One has to keep in mind that the wrong data can quickly turn into a liability.
Furthermore, just plugging in data because it’s available also goes against General Data Protection Regulations (GDPR) principles since Data Owners need to collect only the data they need for their task and nothing more. So Data Scientists need to move away from acting like data plumbers and become data concierges. They have to guide people on the correct data that should be gathered, find the right insights and identify which of that data will drive meaningful outcomes while adhering to strict data governance principles. As you can see, their role is much more expanded than in the past, and so are their responsibilities.
Second, big data is passe! Big data can be defined as data that is produced in large quantities, at high speed, and made up of different types of information. Of course, this doesn’t mean that Big data is no longer critical, but a different kind of data is quickly gaining popularity, and with time, it will become more valuable than Big Data. Here we’re referring to small data, whereby it is estimated that 70% of organizations will shift focus on small data in the coming years. The reasoning behind this is evident; big data is not always available, and many applications have no choice but to rely on small data. The medical domain is one such field, whereby biological information is challenging to collate. Apart from this, more is not always better. In fact, small data can, most of the time, be more insightful than massive datasets. So it’s not about the size but more about the quality of the data. However, we all know that small data has limitations too, which can be overcome using synthetic data. This kind of data has the same features and distribution as small data but is generated in a lab, thus making large datasets available even though there might be a small amount of real-world data. Such data has other benefits too. Since it is created by a machine, synthetic data is not subject to privacy issues. That is why consultancy firms estimate that by 2030, synthetic data will become the most prevailing kind of data used in creating AI models.
Third, the problem with DS is that after analyzing various data streams, we are left with truckloads of insights we will have to process. Traditionally, this was done with Business Intelligence (BI) tools, but most processes are manual and, therefore, unfeasible on a large scale. The solution to this is to use augmented analytics, which uses AI techniques to help prepare and analyze the data. By employing Natural Language Processing algorithms, these insights are further translated from numbers to a human-readable form that is easily understandable by anyone. The system will not only manage snapshots of data but live streams, thus automatically alerting management and guiding them to make quick decisions. By doing so, the dependency on technical personnel is reduced, processes are shortened, and decision-makers are given rapid access to all the required information.
Fourth, the Data Scientist should not be relegated to a data photographer. When management is given a report, it is just a snapshot of the current situation. But situations change rapidly, so information should be live as well. Because of this, a Data Scientist should become a Decision Designer delivering meaningful information at the right time. Automation is critical to achieving this feat. We are all humans and have hundreds of things on our minds, so we need to enact processes that keep us abreast when our world changes. Furthermore, Data Scientists serve a horizontal role that permeates most of the departments within an organization. Thus, they are privy to the big picture, so rather than deliver insights in silos, they should connect the dots and provide management with unified viewpoints.
Fifth, Data Scientists need to be careful when it comes to governance. Due to internal politics, governance varies across diverse styles, from controlling, defensive, and risk-averse to an opposite approach on the other end. All this causes instability which is unfortunate because governance should be simply about deciding how to get things done correctly. Thus, Data Scientists should adopt adaptive governance, a flexible, agile decision-making process that allows fast responses while reducing risks.
The world of DS is passing through rapid changes caused by innovations brought forth by AI. But it’s also an exciting period because an organization can manage to achieve much more with fewer resources and in a shorter time. Thus, adopting these changes is essential, ensuring that the organization reaps all the benefits and places itself ahead of the pack.